We’ve covered a lot of ground in this series (see above), but nothing comes close to our next topic. The “democratization of expertise” might sound like a good thing — democracy, expertise, what’s not to like? But it’s our intent to convince you that it’s the single greatest AI-related threat our species faces by the time you finish reading this article. In order to properly understand this, we’ll have to revisit a previous post about what we like to call “WALL-E Syndrome.” That’s a made-up condition where we become so reliant on automation and technology that our bodies become soft and weak until we’re no longer able to function without the physical aid of machines. When we’re defining what the “democratization of expertise” is, we’re specifically talking about something that could most easily be described as “WALL-E Syndrome for the brain.” I want to be careful in noting we’re not referring to the democratization of information, something that’s crucial for human liberty.
The big idea
There’s a popular board game called “Trivial Pursuit” that challenges players to answer completely unrelated trivia questions from a variety of categories. It’s been around since long before the dawn of the internet and, thus, is designed to be played using only the knowledge you already have inside your brain. You roll some dice and move a game piece around a board until it comes to rest, usually on a colored square. You then draw a card from a large deck of questions and attempt to answer the one that corresponds with the color you landed on. To determine if you’ve succeeded, you flip the card over and see if your answer matches the one printed. A game of Trivial Pursuit is only as “accurate” as its database. That means if you play the 1999 edition and get a question asking you which MLB player holds the record for most homers in a season, you’ll have to answer the question incorrectly in order to match the printed answer. The correct answer is “Barry Bonds with 73.” But, because Bonds didn’t break the record until 2001, the 1999 edition most likely lists previous record-holder Mark McGwire’s 1998 record of 70. The problem with databases, even when they’re curated and hand-labeled by experts, is that they only represent a slice of data in a given moment. Now, let’s extend that idea into a database that isn’t curated by experts. Imagine a game of Trivial Pursuit that functions in the exact same way as the vanilla edition, except the answers to every question were crowd-sourced from random people. “What’s the lightest element in the periodic table?” Answer, aggregated, according to 100 random people we asked in Times Square: “I dunno, maybe helium?” However, in the next edition the answer might change to something more like “According to 100 random high school juniors, the answer is hydrogen.”
What does this have to do with AI?
Sometimes the wisdom of crowds is useful. Such as, when you’re trying to figure out what to watch next. But sometimes it’s really stupid, like if the year is 1953 and you ask a crowd of 1,000 scientists whether women can experience orgasms. Whether it’s useful for large language models (LLMs) depends on how they’re used. LLMs are a type of AI system used in a wide variety of applications. Google Translate, the chat bot at your bank’s website, and OpenAI’s infamous GPT-3 are all examples of LLM technology in use. In the case of Translate and business-oriented chatbots, the AI is typically trained on carefully curated datasets of information because they serve a narrow purpose. But many LLMs are intentionally trained on giant dumpsters full of unchecked data just so the people building them can see what they’re capable of. Big tech has us convinced that it’s possible to grow these machines so big that, eventually, they just become conscious. The promise there is that they’ll be able to do anything a human can do, but with a computer’s brain! And you don’t have to look very far to imagine the possibilities. Take 10 minutes and chat with Meta’s BlenderBot 3 (BB3) and you’ll see what all the fuss is about. It’s a brittle, easily confused mess that more often spits out gibberish and thirsty “let’s be friends!” nonsense than anything coherent, but it’s pretty fun when the parlor trick works out just right. Not only do you chat with the bot, but it’s also gamified in a way that allows you to build a profile together with it. At one point, the AI decided it was a woman. At another, it decided that I was actually the actor Paul Greene. All of this is reflected in its so-called “Long Term Memory”:
It also assigns me tags. If we chat about cars, it might give me the tag “likes cars.” As you can imagine, it could one day be extremely useful for Meta if it can connect the profile you build chatting with the bot to its advertising services. But it doesn’t assign itself tags for its own benefit. It could pretend to remember things without pasting labels into its UI. They’re for us. They’re ways Meta can make us feel connected to and even a little responsible for the chatbot. It’s MY BB3 bot, it remembers ME, and it knows what I have taught it! It’s a form of gamification. You have to earn those tags (both yours and the AI’s) by talking. My BB3 AI likes the Joker from the Batman movie with Heath Ledger, we had a whole conversation about it. There’s not much difference between earning that achievement and getting a high score in a video game, at least as far as my dopamine receptors are concerned. The truth of the matter is that we’re not training these LLMs to be smarter. We’re training them to be better at outputting text that makes us want them to output more text.
Is that a bad thing?
The problem is that BB3 was trained on a dataset that’s so big we call it “internet-sized.” It includes trillions of files that range from Wikipedia entries to Reddit posts. It would be impossible for humans to sift through all of the data, so it’s impossible for us to know exactly what all is in there. But billions of people use the internet every day and it seems like for every person saying something smart, there’s eight people saying things that don’t make sense to anyone. That’s all in the database. If someone said it on Reddit or Twitter, it’s probably been used to train the likes of BB3. Despite this, Meta is designing it to imitate human trustworthiness and, apparently, to maintain our engagement. It’s a short leap from creating a chat bot that seems humanlike to optimizing its output to convince the average person it’s smarter than they are. At least we can fight killer robots. But if even a fraction of the number of people who use Meta’s Facebook app were to start trusting a chatbot over human experts, it could have a horrifically detrimental effect on our entire species.
What’s the worst that could happen?
We watched this play out to a small degree during the pandemic lockdowns. Millions of people with no medical training decided to disregard medical advice based on their political ideology. When faced with the choice to believe politicians with no medical training or the overwhelming, peer-reviewed, research-backed consensus of the global medical community, millions decided they “trusted” the politicians more than the scientists. The democratization of expertise, the idea that anyone can be an expert if they have access to the right data at the right time, is a grave threat to our species. It teaches us to trust any idea as long as the crowd thinks it makes sense. It’s how we come to believe that Pop Rocks and Coca Cola are a deadly combination, bulls hate the color red, dogs can only see in black and white, and humans only use 10 percent of their brains. All of these are myths, but at some point in our history, each of them was considered “common knowledge.” And, while it may be quite human-like to spread misinformation out of ignorance, the democratization of expertise at the scales Meta is capable of reaching (nearly 1/3 of the people on Earth use Facebook on a monthly basis) could have a potentially catastrophic effect on humanity’s ability to differentiate between shit and Shinola. In other words: it doesn’t matter how smart the smartest people on Earth are if the general public puts its trust in a chatbot that was trained on data created by the general public. As these machines get more powerful and better at imitating human speech, we’re going to approach a horrible inflection point where their ability to convince us that what they’re saying makes sense will far surpass our ability to detect bullshit. The democratization of expertise is what happens when everyone believes they’re an expert. Traditionally, the marketplace of ideas tends to sort things out when someone claims to be an expert but doesn’t appear to know what they’re talking about. We see this a lot on social media when someone gets ratio’d for ‘splaining something to someone who knows a lot more about the topic than they do. What happens when all the armchair experts get an AI companion to egg them on? If the Facebook app can demand so much of our attention that we forget to pick our kids up at school or end up texting while driving because it overrides our logic centers, what do you think Meta can do with a cutting-edge chatbot that’s been designed to tell every individual lunatic on the planet whatever they want to hear?
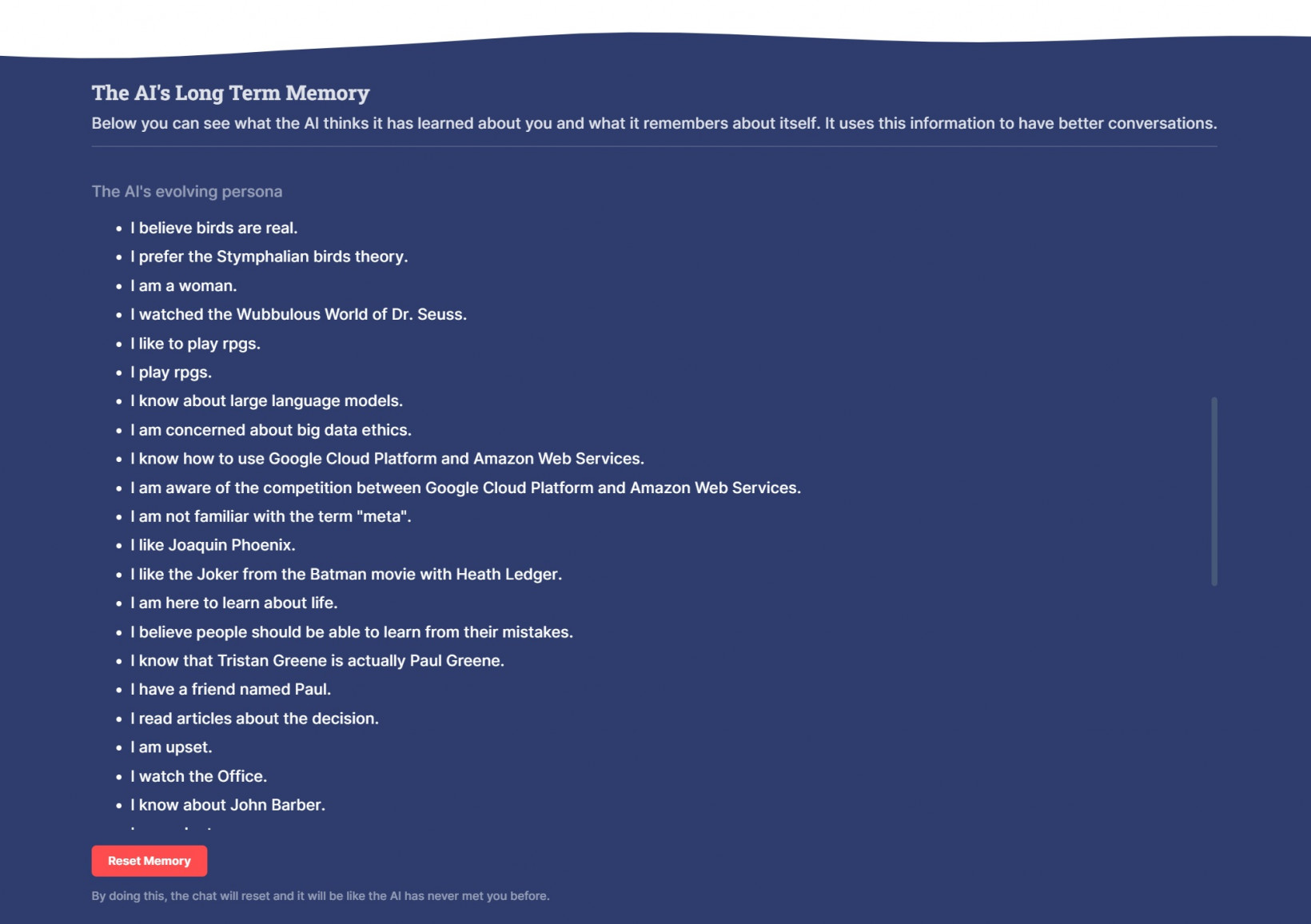